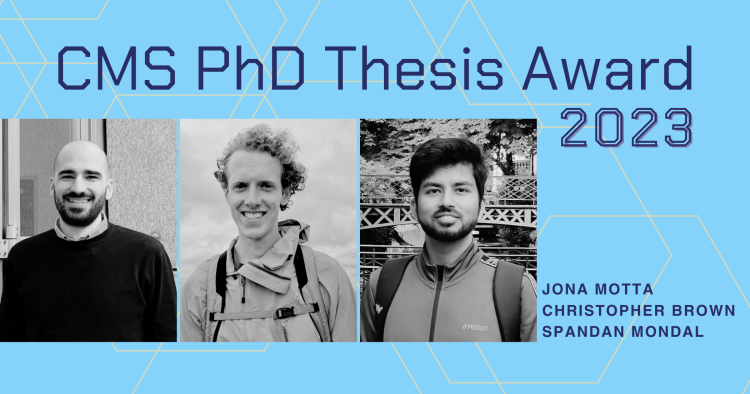
The CMS Collaboration is thrilled to announce that laureates of the 2023 CMS PhD Thesis Award are Jona Motta, Christopher Edward Brown, and Spandan Mondal. This award recognizes exceptional doctoral research within the collaboration that pushes the boundaries of high-energy physics. Out of a highly competitive pool of 27 nominees, this year's winners impressed the CMS Thesis Award Committee with their outstanding work.
“Doctoral students do a lot of impressive work in CMS. Writing a PhD thesis to document this work is a tremendous effort and achievement. Some of the students decide to invest substantial extra efforts in writing an exceptionally clear, effective and original documentation of their research work. They write for their peers, future students, who will follow in their steps, and for all those in search of in-depth, detailed, accurate but also accessible knowledge related to CMS scientific or technical frontline research. The CMS Thesis Award recognizes the result of these exceptional efforts.” - Marta Felcini, chair of the CMS PhD Thesis Award Committee.
Now, let's get to know the winners and their insightful research...
Jona Motta - LLR, Institut Polytechnique de Paris
My thesis “Development of machine learning based τ trigger algorithms and search for Higgs boson pair production in the bbττ decay channel with the CMS detector at the LHC” was conducted at a very exciting time (2020-2023): at the crossroads of past, present, and future. I had the chance to work on the analysis of the data recorded with the CMS detector during the Run-2 of the LHC (2016-2018), to contribute to the commissioning of the CMS Level-1 Trigger for the Run-3 data-taking (2022-2025), and to develop an innovative Level-1 Trigger algorithm for the CMS Phase-2 upgrade (2029-onwards).
The focal point of my Thesis is the search for Higgs boson pairs production, which is the only process giving direct access to a very interesting property of the Higgs boson: its self-coupling (i.e. its ability to interact with itself). This is one of the last missing pieces of the Standard Model of particle physics, and its measurement would amount to a closure test of the Standard Model itself. In my Thesis I present stringent limits on the Higgs boson self-coupling and its deviations from the expected value due to possible new physics processes. These results are obtained analysing the CMS Run-2 data and searching for Higgs boson pair production in the final state where one boson decays to two bottom quarks and the other to two τ leptons.
As the LHC operations restarted in 2022, the CMS experiment needed to be commissioned to achieve a successful Run-3 data-taking. In this context, the Thesis presents my contributions to the optimisation and commissioning of the Level-1 trigger algorithms reconstructing τ leptons, electrons, and photons. Moreover, my Thesis details the design of a new and powerful machine learning technique, called Calibraton, for the calibration of calorimeter inputs to the Level-1 trigger.
To further probe the SM, with particular interest in the Higgs boson self-coupling, the High-Luminosity LHC (HL-LHC) operations are scheduled to start in 2029. To achieve the best performance of future analyses involving τ leptons, in my Thesis I developed a novel τ triggering algorithm called TauMinator, which casts the Level-1 trigger τ lepton reconstruction into an image recognition problem using Convolutional Neural Networks designed to run on state-of-the-art FPGAs (Field Programmable Gate Arrays).
In the coming years, I will continue contributing to the Higgs pair productions searches, in the hope of reaching the first ever measurement of the Higgs boson self-coupling; alongside this I hope to contribute to the development of ever better analysis strategies involving machine learning for the characterisation of the Higgs sector and possibly the discovery of exciting new physics awaiting for us in the data being collected at CMS.
Christopher Brown - Imperial College London
My thesis “Fast Machine Learning in the CMS Level-1 Trigger for the High-Luminosity LHC” was for the upgrade of CMS ready for the high-luminosity LHC, where I was involved in the upgrade to the Level-1 trigger. My work focussed on implementing machine learning algorithms within the Level-1 trigger in order to boost its performance. Any events not triggered on at Level-1 are lost forever so making sure the trigger is as effective as possible is highly important. The first algorithm I helped implement was using gradient boosted decision trees to evaluate the quality of tracks being produced by the Level-1 track finding algorithm which allows downstream algorithms to remove poorly reconstructed tracks that were impacting their efficiency. The second algorithm I implemented was a three-part neural network for primary vertex finding and associating tracks to this vertex which is essential for reducing the inefficiency due to additional overlapping proton-proton interactions in every LHC collision.
The final part of my thesis was focussing on the impact of a dynamic environment for machine learning in the trigger. CMS is not a static environment; the beam conditions from the LHC are constantly changing and the detector itself is not perfectly functioning and efficient at all times. I showed that dynamically updating machine learning models as detector conditions change not only allowed their performance to improve relative to no retraining but also allowed the models to become more robust to the changing conditions as they learnt to ignore background variation in their training data. These techniques have the potential to improve the CMS trigger over the lifetime of the experiment and make sure that our trigger algorithms are as performant as possible.
My future plans are to continue my work in the CMS Level-1 trigger upgrade as part of the Next Generation Trigger project where I will continue to implement advanced AI algorithms into the CMS Level-1 trigger and also focus on machine learning operations for the wide array of algorithms being planned.
Spandan Mondal - RWTH Aachen University
The Higgs boson decaying to a charm quark-antiquark pair is a particularly interesting process, as its rate can be significantly altered by the presence of new physics beyond the Standard Model, due to the small mass (~1.3 GeV) of the charm quark. However, the small mass of the charm quark also makes this process extremely rare. Moreover, identifying charm quarks at the CMS experiment is difficult, making the search extremely challenging.
In my thesis, “Charming decays of the Higgs, Z, and W bosons: Development and deployment of a new calibration method for charm jet identification” I developed a novel calibration algorithm for charm jet identification that enables maximal use of the available information related to jets arising from charm quarks. The new method is used to correct the entire distribution expected as output when jet flavour identification algorithms are applied to jets of different flavours. The calibrated results improve upon traditional efficiency measurements and help enhance the sensitivities of searches involving charm quarks.
The second part of my thesis focuses on analysing data collected by the CMS experiment to search for events where a Higgs boson, produced in association with a leptonically-decaying Z or W boson, decays to a charm quark-antiquark pair. The charm quarks are reconstructed using two separate (“resolved”) small-radius jets whose flavours are predicted using modern machine-learning (ML) based flavour tagging algorithms calibrated using the aforementioned novel method. Upon a statistical combination with a complementary merged-jet-based search, an upper limit on the process of interest is obtained, which is the most stringent direct limit to date. The analysis is validated by studying the decay of the Z boson into charm quarks and the corresponding process is observed for the first time at a hadron collider experiment. I further extended my thesis by studying the decay of W bosons into charm-strange quark pairs, leveraging correlated tagging of charm and strange jets. This marked the first observation of charmed W boson decays in the diboson channel at a hadron collider experiment.
Moving on, I am currently working on developing new ML-based reconstruction and calibration methods for identifying heavy-flavour hadronic decays. I hope to extend the use of novel data analysis techniques across a broad range of studies within the CMS collaboration.