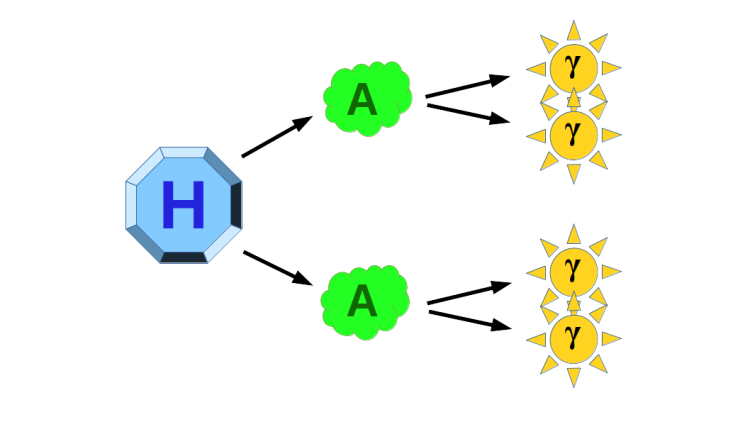
While experimental results at the LHC continue to show good agreement with the standard model (SM), which is currently the best theory of fundamental particle interactions, the CMS physicists continue to turn up the heat on the SM. In fact, it is generally accepted that the SM cannot be the final theory of particle physics since it leaves unanswered many important questions, such as the nature of dark matter. As a theory of particle physics, the SM makes precise predictions about what we should see, or not, in the LHC experiments. The CMS physicists test these predictions by making equally precise measurements, using collision data, and by searching for any deviations between the experimental results and the theoretical predictions. In this way, physicists hope to find where the SM departs from reality and then use this information to develop a better theory.
However, there are many challenges on the path leading to the discovery of “new physics” at the LHC. For example, maybe some signals will not be observable until we reach higher collision energies than those available at the LHC. Or maybe we are not efficient enough in detecting potential new physics signals in the collected data. In particular, a number of recent theories of new physics predict decays that may be challenging to reconstruct using standard reconstruction algorithms. Such “exotic decays” may remain hidden among more conventional particles or be so odd that they are rejected from the analysis event samples. In recent years, CMS physicists have developed new analysis procedures to improve the probability of finding such new physics signals, if they are produced in the LHC collisions.
Although machine learning (ML) techniques were used in particle physics since the 1980’s, and in CMS since its birth, these have traditionally relied on the highly processed inputs produced by reconstruction algorithms rather than on the more basic “raw” data provided by the particle detectors. This data analysis paradigm was instrumental in several CMS physics analyses, including some interesting discoveries, but was not good enough to overcome the challenges posed by many exotic decays. In fact, the limiting factor was not so much the performance of the ML algorithms themselves but rather of the algorithms used to turn the raw detector data into basic physics observables.
Enter modern artificial intelligence (AI) and deep learning. Thanks to this newer breed of ML algorithms, it is now possible to bypass the conventional reconstruction step and directly process the detector data. Applying AI to directly extract particle information from low-level detector data (a new approach often referred to as end-to-end particle reconstruction) promises to unlock potential information pathways that could solve some of the biggest challenges we face when searching for exotic decays.
CMS physicists have just shown, for the first time, how such an end-to-end particle reconstruction strategy can be used to successfully perform a new physics measurement, one that would have been impossible to do using previous approaches. They re-examined events resembling the diphoton decay of the Higgs boson (H→γγ), one of the pillars of the Higgs boson discovery in 2012. These events provided pivotal evidence that the new particle behaved just like the Higgs boson predicted by the SM. However, according to a number of theories of new physics, similar event signatures could be caused by an axion-like particle (ALP or just A), which could be a potential dark matter candidate. If the Higgs boson were allowed to decay into a pair of these hypothetical ALPs (H→AA), and if the ALPs were light enough, each ALP would have high momentum (“boost”) and thus decay into a highly overlapping or “merged” pair of photons (A→γγ).
From the point of view of the CMS detector, these A→γγ decays would be nearly indistinguishable from the signature of a single photon, as illustrated in Fig. 1 for different degrees of merging. Therefore, this type of exotic decay would go completely unnoticed when probed with standard algorithms: it would be hidden in plain sight among events categorized as H→γγ. By using a new end-to-end particle reconstruction technique, the CMS physicists achieved a breakthrough in the detection of these merged ALP decays and performed the first-ever direct search for H→AA, A→γγ decays in the CMS events.
![]() |
![]() |
![]() |
Fig 1. The energy signature of a single A→γγ decay as it would be seen by the CMS ECAL. The energy deposits are indexed by their positions in azimuth (ϕ) and pseudorapidity (η). For ALP masses of mA = 1.0 GeV (left), the two photon clusters are separated but begin to overlap. This merging makes it difficult to distinguish the A→γγ energy pattern from that of a single photon. For mA = 0.4 GeV (middle), the overlap is even stronger, though distinct cluster maxima remain visible. At mA = 0.1 GeV (right), the merging is such that the two photons land in the same crystal. These fully overlapping energy clusters are impossible to distinguish from the cluster of a single photon, except through the use of AI.
Although the study found no evidence of any ALP decays, it set the most stringent constraints to date on the possible production of these particles. The black dots and solid line in Fig. 2 show the measured CMS upper limit on the H→AA →4γ decay rate as a function of the mass mA of the A particle, using data collected in the 2016–2018 period. This new end-to-end reconstruction technique sets a standard for what is possible at the LHC using modern AI.
Fig. 2: Observed (black solid line) and expected (blue dashed line) upper limit at 95% confidence level on the Higgs boson decay rate for the process H→AA →4γ as a function of the mass mA of the hypothetical particle A. The green and yellow bands represent the 68% and 95% confidence intervals of the expected upper limit, respectively.
As CMS researchers continue to probe the capabilities of AI, they see that the achieved improvements can be quite significant. By re-examining the full data analysis workflow with an eye for the strengths of AI, substantial performance gains are achievable, opening the door to several measurements and searches. As CMS scientists continue to push the boundary of new physics searches, artificial intelligence will no doubt be a powerful tool to peer through our particle detectors.
Read more about these results:
-
CMS Paper "Search for exotic Higgs boson decays H with events containing two merged diphotons in proton-proton collisions at 13 TeV"
-
@CMSExperiment on social media: facebook - twitter - instagram
- Do you like these briefings and want to get an email notification when there is a new one? Subscribe here