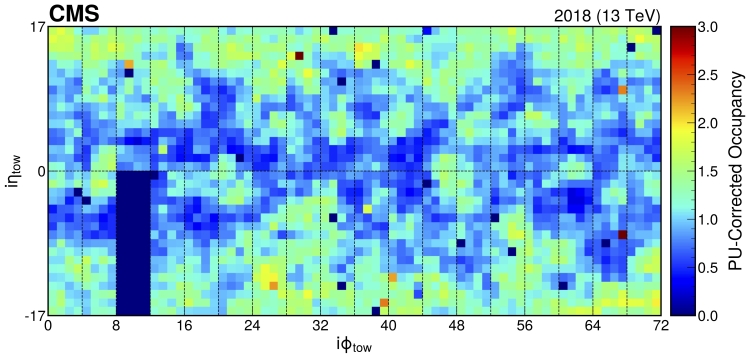
CMS develops and deploys a new machine-learning technique based on neural networks that is able to spot existing and developing anomalies in the functioning of the detector.
In the quest to uncover the fundamental particles and forces of nature, one of the critical challenges facing high-energy experiments such as CMS, is ensuring the quality and high fidelity of the vast amounts of data collected. The electromagnetic calorimeter (ECAL), a crucial component of the CMS detector, measures the energy of particles, mainly electrons and photons, produced in collisions of protons at the LHC. Ensuring the accuracy and reliability of recorded data is paramount for the successful operation of an experiment to make groundbreaking discoveries like the Higgs boson.
CMS researchers have developed and deployed an innovative machine learning (ML) technique to enhance the current Data Quality Monitoring (DQM) system of ECAL during Run 3 of the LHC, currently ongoing. Detailed in a recent publication, this new approach promises to improve the accuracy and efficiency of detecting anomalies in both the spatial and temporal domains of online data streams. Such real-time capability is essential in the fast-paced LHC environment for the timely detection and correction of detector issues.
An image from ECAL data with anomalous regions (left) which when passed to the ML- system produces the easily identifiable colour map on the right, showing anomalous regions in red and good regions in green.
The traditional CMS DQM consists of conventional software that relies on a combination of predefined rules, thresholds, and manual inspections to alert humans in the control room of potential detector issues. This approach involves setting specific criteria for what constitutes 'normal' data behaviour and flagging deviations. While effective, these methods can potentially miss subtle or unexpected anomalies that don't fit predefined patterns.
In contrast, the novel ML-based system learns the normal detector behaviour from existing good data that it is trained on and then detects any deviations. The cornerstone of the ML approach is an autoencoder-based anomaly detection system. Autoencoders, a specialized type of neural network, are designed for unsupervised learning tasks.
The system, fed with ECAL data in the form of 2D images, is also adept at spotting anomalies that evolve over time thanks to novel correction strategies. This temporal aspect is crucial for recognizing patterns that may not be immediately apparent but develop gradually.
The novel autoencoder-based system not only bolsters the performance of the CMS detector but also serves as a model for real-time anomaly detection across various fields, highlighting the transformative potential of artificial intelligence. For example, industries that manage large-scale, high-speed data streams, such as finance, cybersecurity, and healthcare, could benefit from similar ML-based systems for anomaly detection, enhancing their operational efficiency and reliability.
Learn more about the research in the related paper: Autoencoder-Based Anomaly Detection System for Online Data Quality Monitoring of the CMS Electromagnetic Calorimeter