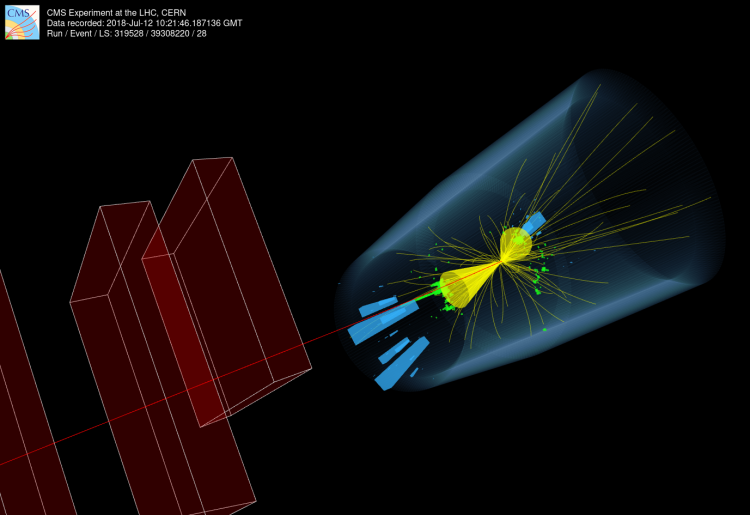
Very quickly after quarks are produced in proton-proton collisions, they radiate gluons (the mediators of the strong force between quarks) that in turn produce more particles that radiate gluons. This creates an avalanche of particles in the detector. At some point the energy of the particles becomes too low to radiate further particles, and the leftover quarks form quark bound states known as hadrons. Therefore, a quark produces a collimated spray of energetic particles in the detector which we call a jet.
Jets are a signature of many interesting processes at the LHC. For example, the Higgs boson often decays into b or c quark-antiquark pairs. Such pairs may also appear in the decay of hypothetical particles, suggested in new theories. Typically, this would result in two jets. However, an interesting topology arises if the particle decaying into a quark-antiquark pair is “boosted”, i.e. produced with high energy [see an example here]. The two jets start overlapping and instead of two jets, one large jet is seen in the detector, as illustrated in Fig. 1
Figure 1: If a decaying boson is given enough boost, the quark-antiquark pairs become collimated and the two jets merge into a large jet. Sketch taken from here
Given the important role that jets containing two b or c quarks play in understanding the Higgs boson and/or in looking for new phenomena, it is crucial to correctly identify them in the detector. This is known as jet “flavour tagging”, which in this case means to know if the observed large jet originates from a pair of b (double-b jet), c (double-c jet), or lighter quarks, or even from a gluon.
Knowing the differences between the hadrons of different kinds (flavours) is a key factor in this challenging task. In particular, unlike hadrons from other quarks, b and c hadrons travel relatively long distances before decaying. This is seen in our detector as a presence of tracks whose intersection, called vertex, is displaced from the location of the proton-proton collisions. We can then require our jets to have two displaced vertices in an attempt to preferably select double-b or double-c jets. In reality, we also use many other features in determining the jet flavour.
The flavour tagging has evolved from manually imposing conditions on the jet properties to machine learning techniques and, more recently, to complex state-of-the-art “deep learning” algorithms such as ParticleNet. One of the strengths of these techniques is the ability to recognize the sophisticated correlations between the multitude of physical observables and exploit them to achieve the best precision in correctly identifying the jet flavours. This has resulted in a steady and significant improvement in performance over the years.
To train the machine learning algorithms, we simulate collisions that produce jets of different flavours. In simulation, we know the particle content of each jet and therefore we can quantify how well the flavour tagging algorithms perform. However, simulations are imperfect. Is the true performance in collision data the same as that in our simulations? The challenge in answering this is selecting events in the data that are rich in double-b or double-c jets, called signal jets, because the other types of jets are far more numerous. We achieved this in three different ways and as soon as this challenge is overcome, one can correct the performance in simulation using that of the data.
This first method uses Z boson (carrier of the weak force) decays to quark-antiquark pairs for calibration. It selects events that have a large energetic jet with mass close to the mass of the Z boson. However, many other standard model processes produce events with energetic jets, manifested as a large background in the measurement. This makes the method less sensitive than the other two. It is used only to calibrate the double-b algorithms because the reduced sensitivity makes it unsuitable for calibrating the double-c algorithms.
Figure 2: Display of a potential Z→bb event recorded by CMS in 2018. You can open the interactive event display on this separate page.
The other two methods are more precise because they use jets originating from gluons, which are the most numerous jets created in proton-proton collisions. The downside is that, generally, gluon jets and jets originating from heavy particles are different. Hence, the flavour tagging performances of the same algorithm for the two kinds of jets are not the same. We therefore carefully select a special subset of gluon jets, those that are likely to have split into a pair of b or c quarks. The properties of these "proxy jets" resemble those of signal jets.
One of the two proxy jet methods works with jets that contain a muon. Since muons appear more often in b and c jets than in the jets coming from the lighter quarks, this requirement enriches the selected jets with double-b and double-c jets. The method also requires a selection on the jet content, considering only those jets that are likely to have originated from two energetic particles, making the proxy jets more similar to double-b and double-c jets.
The second proxy jet method is a novel method that employs a machine learning technique, called a boosted decision tree (BDT), and trains it on simulated jets in order to select proxy jets in data. In the training process, proxy jets are defined as those likely to contain two b or c quarks, as illustrated in Fig. 3, as opposed to jets that are primarily composed of energetic gluons. This ensures the similarity of proxy and signal jets, but special efforts have been made to evaluate the effects of the BDT selection on the final result.
Figure 3: Illustration of a gluon-initiated jet with properties similar to the signal jets. Such jets are selected in the proxy method using a boosted decision tree.
The results of the three methods are statistically combined to obtain the best estimate of the performance of the tagging algorithms with an excellent precision. The reduced uncertainties on the tagging performance will greatly enhance the sensitivity of physics measurements that use these algorithms. This result also provides a glimpse of the efforts that go into the development and validation of advanced algorithms used in various physics measurements at the CMS experiment.
Matteo Marchegiani, Sen Deng, Congqiao Li, and Matej Roguljic are the PhD students at the time who have played a key roles in the collaborative effort of developing the three calibration methods and statistical combination framework.
Read more about these results:
-
CMS Physics Analysis Summary (BTV-22-001): "Performance of heavy-flavour jet identification in boosted topologies in proton-proton collisions at 13 TeV"
-
@CMSExperiment on social media: LinkedIn - facebook - twitter - instagram
- Do you like these briefings and want to get an email notification when there is a new one? Subscribe here