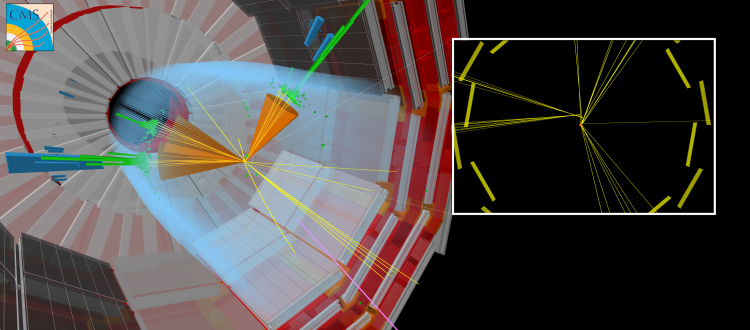
Nowadays, artificial neural networks have an impact on many areas of our day-to-day lives. They are used for a wide variety of complex tasks, such as driving cars, performing speech recognition (for example, Siri, Cortana, Alexa), suggesting shopping items and trends, or improving visual effects in movies (e.g., animated characters such as Thanos from the movie Infinity War by Marvel).
Traditionally, algorithms are handcrafted to solve complex tasks. This requires experts to spend a significant amount of time to identify the optimal strategies for various situations. Artificial neural networks - inspired by interconnected neurons in the brain - can automatically learn from data a close-to-optimal solution for the given objective. Often, the automated learning or “training” required to obtain these solutions is “supervised” through the use of supplementary information provided by an expert. Other approaches are “unsupervised” and can identify patterns in the data. The mathematical theory behind artificial neural networks has evolved over several decades, yet only recently have we developed our understanding of how to train them efficiently. The required calculations are very similar to those performed by standard video graphics cards (that contain a graphics processing unit or GPU) when rendering three-dimensional scenes in video games. The ability to train artificial neural networks in a relatively short amount of time is made possible by exploiting the massively parallel computing capabilities of general-purpose GPUs. The flourishing video game industry has driven the development of GPUs. This advancement, along with the significant progress in machine learning theory and the ever-increasing volume of digitised information, has helped to usher in the age of artificial intelligence and “deep learning”.
In the field of high energy physics, the use of machine learning techniques, such as simple neural networks or decision trees, have been in use for several decades. More recently, the theory and experimental communities are increasingly turning to the state-of-the-art techniques, such as “deep” neural network architectures, to help us understand the fundamental nature of our Universe. The standard model of particle physics is a coherent collection of physical laws - expressed in the language of mathematics - that govern the fundamental particles and forces, which in turn explain the nature of our visible Universe. At the CERN LHC, many scientific results focus on the search for new “exotic” particles that are not predicted by the standard model. These hypothetical particles are the manifestations of new theories that aim to answer questions such as: why is the Universe predominantly composed of matter rather than antimatter, or what is the nature of dark matter?
Recently, searches for new particles that exist for more than a fleeting moment in time before decaying to ordinary particles have received particular attention. These “long-lived” particles can travel measurable distances (fractions of millimetres or more) from the proton-proton collision point in each LHC experiment before decaying. Often, theoretical predictions assume that the long-lived particle is undetectable. In that case, only the particles from the decay of the undiscovered particle will leave traces in the detector systems, leading to the rather atypical experimental signature of particles apparently appearing from out of nowhere and displaced from the collision point.
Figure 1: Schematic of the network architecture. The upper (orange and blue) sections of the diagram illustrate the components of the network that are used to distinguish jets produced in the decays of long-lived particles from jets produced by other means, trained with simulated data. The lower (green) part of the diagram shows the components that are trained using real collision data (see text for details).
Standard algorithms used to interpret the data from proton-proton collisions are not designed to seek out such odd-looking events. Therefore, the CMS Collaboration has developed an artificial neural network to identify these unusual signatures. The neural network has been trained (with supervision) to distinguish sprays of particles known as “jets” produced by the decays of long-lived particles from jets produced by far more common physical processes. A schematic of the network architecture is shown in Fig. 1 and its performance is illustrated in Fig. 2. The network learns from events that are obtained from a simulation of proton-proton collisions in the CMS detector.
Figure 2: An illustration of the performance of the network. The coloured curves represent the performance of different theoretical supersymmetric models. The horizontal axis gives the efficiency for correctly identifying a long-lived particle decay (i.e. the true-positive rate). The vertical axis shows the corresponding false-positive rate, which is the fraction of standard jets mistakenly identified as originating from the decay of a long-lived particle. As an example, we use a point of the red curve where the fraction of genuine long-lived particles that are correctly identified is 0.5 (i.e. 50%). This method misidentifies only one regular jet in every thousand mistakenly as originating from a long-lived particle decay.
A novel aspect of this study involves the use of data from real collision events, as well as simulated events, to train the network. This approach is used because the simulation - although very sophisticated - does not exhaustively reproduce all the details of the real collision data. In particular, the jets arising from long-lived particle decays are challenging to simulate accurately. The effect of applying this technique, dubbed "domain adaptation," is that the information provided by the neural network agrees to a high level of accuracy for both real and simulated collision data. This behaviour is a crucial trait for algorithms that will be used by searches for rare new-physics processes, as the algorithms must demonstrate robustness and reliability when applied to data.
The CMS Collaboration will deploy this new tool as part of its ongoing search for exotic, long-lived particles. This study is part of a larger, coordinated effort across all the LHC experiments to use modern machine techniques to improve how the large data samples are recorded by the detectors and the subsequent data analysis. For example, the use of domain adaptation may make it easier to deploy robust machine-learned models as part of future results. The experience gained from these types of study will increase the physics potential during Run 3, from 2021, and beyond with the High Luminosity LHC.
Figure 3: Histograms of the output values from the neural network for real (black circular markers) and simulated (coloured filled histograms) proton-proton collision data without (left panel) and with (right panel) the application of domain adaptation. The lower panels display the ratios between the numbers of real data and simulated events obtained from each histogram bin. The ratios are significantly closer to unity for the right panel, which indicates an improved understanding of the neural network performance for real collision data, which is crucial to reduce false positive (and false negative!) scientific results when searching for exotic new particles.
Read more about these results in the CMS Physics Analysis Summaries:
- Log in to post comments