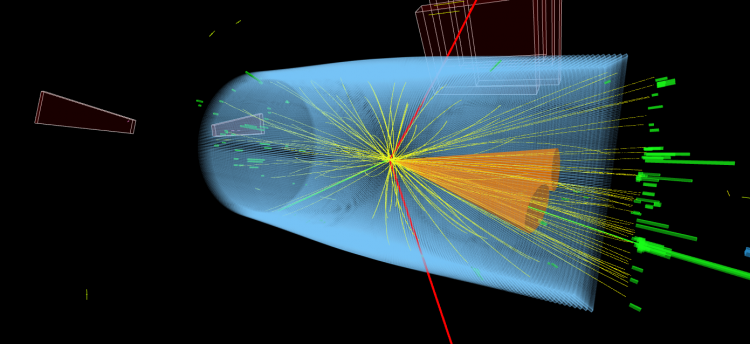
Using machine learning to improve the detection of new physics in the interactions of the top quark
Although our theory describing the interactions between fundamental particles is exceptionally successful, it has weaknesses. You may have heard, for instance, the existence of dark matter or the fact that neutrinos have mass, which are two proven phenomena we cannot yet explain. That is why physicists are relentlessly searching for new types of particles or interactions to improve our incomplete theory in a quest to solve some of the deepest mysteries of our Universe.
But what if new particles are simply too massive to be produced even at the LHC, the most powerful accelerator ever built? Fortunately, the laws of the quantum world make it possible to detect them indirectly. According to Heisenberg’s uncertainty principle, even if the energy of a collision is insufficient to generate the mass of a heavy particle, there is still a small probability that it will briefly appear and then disappear during the interaction. In the rare cases when this happens, some properties of the other particles involved in the interaction will be modified. In practice, if new massive particles really exist, they would create tiny deviations in our measurements with respect to the predictions.
Then, the remaining question is “which measurements should we perform to look for these deviations”? There exist many proposed extensions to our theory. While the predictions of the most promising ones are directly put to the test, it is impossible to look in all directions at once and to track all the imaginable anomalies that could hide in our data. For this reason, an approach known as effective field theory is being used more and more frequently at the LHC, in which the results are expressed in terms of generic classes of new physics effects. These results can later be compared to the predictions of any given theoretical extension to check whether it is supported by the data or can be ruled out. This approach makes it possible to relate potential anomalies coherently observed in different, independent, measurements and interpret them as being caused by massive unknown particles. With increasing precision, we can then pinpoint better the properties of these particles and determine how they interact with others. This is thus a valuable tool for physicists to make the most out of the LHC data and maximize our chances of finding “something” new. A qualitative description of this approach can also be found in this previous briefing.
A new CMS analysis used an effective field theory to search for anomalies in how the top quark interacts with the Z boson. Discovered about 25 years ago, the top quark is one of the most intriguing elementary particles, notably because its mass is significantly larger than all the others. This has many profound implications, such as a privileged coupling to the Higgs boson and possibly to new particles. The LHC is currently the only accelerator in the world that can produce top quarks copiously, and they are being studied in every detail there. Because it requires so much energy to produce a top quark in association with a Z boson (which is about half as heavy), the interaction between these particles was not yet tested with the same precision as other top quark couplings. With this new analysis, we aim to probe this interaction as finely as possible to verify whether we measure a value sensibly different than what is predicted.
The key feature of this work lies in the use of novel machine-learning techniques to improve the measurement. Machine learning is becoming ubiquitous in our society, from facial recognition to self-driving cars. The same is happening in high-energy physics, where such algorithms can help us find the needle (for instance, feeble signs of new physics) in the haystack (piles of data that mostly contain known phenomena). During the so-called “training phase”, the parameters of these algorithms inspired from the biological structure of a brain get adjusted until a mathematical function is found that is able to perform a complex task with better performance than simpler algorithms programmed explicitly by humans. In this analysis, we trained deep neural networks with simulated collisions and made them learn the characteristics of the types of signals we are searching for in the data. A first neural network was tasked with recognizing collisions that are most likely to have produced a top quark and a Z boson together. Once these most interesting collisions got selected, another neural network learned to identify potential anomalies in the CMS detector that would indirectly signal the existence of new particles. It is the first time that an analysis exploits such techniques to look for signs of new physics in the context of an effective field theory. The main challenge was to make the algorithms learn to detect very broad classes of anomalies without relying on any simplifying assumptions.
Figure 1: A plot comparing the number of interesting collisions observed in the CMS data, shown as black dots, or predicted by the theory, shown as colored histograms. The collisions are categorized by a neural network according to the probability that they contain anomalies in the interaction of the top quark with the Z boson, increasing from left to right. The moving dotted line shows that, when the magnitude of some anomalous effect increases, more and more collisions get categorized as being abnormal by the neural network. The bottom panel shows the ratio of the number of collisions that we would then expect to observe compared to what is predicted by the current theory.
Then, among the collisions - also called events - observed by CMS that were considered interesting for this work, the next step was to ask our neural network how many of them looked like they had involved new particles. This is illustrated in Figure 1, where the black dots representing our data are compared with colored histograms representing our predictions. From left to right, the quantity on the x-axis indicates whether the neural network is confident that the collisions are completely normal, or on the contrary that they look like they may contain some anomalies. The moving dotted line shows how the targeted events would get categorized by the neural network when increasing the magnitude of some type of anomaly affecting the coupling of the top quark to the Z boson. The bottom panel shows the ratio of the number of events that we would then expect to observe compared to what is predicted by the current theory. One can see that the stronger these anomalies, the more collisions would be categorized as abnormal by the neural network, which would be a clear indication that we are on the track of something new. However, the data points are in very good agreement with the predictions. This agreement either means that there is nothing new hiding in the specific data, or that the magnitude of the effect is too small for particle physicists to detect with the current precision.
Figure 2: A plot showing the level of agreement of the data for ranges of magnitudes of two types of anomalies represented in the x and y axes. Our theory predicts that both magnitudes are exactly equal to zero. The red cross indicates that the data are in very good agreement with this hypothesis. Magnitudes of these anomalies that lie outside the red ellipse are not supported by the data and can be excluded with a high level of confidence.
The x and y axes of Figure 2 show possible magnitudes for two types of anomalies, and the color code indicates the level of agreement of the data with each hypothesis. Since our theory does not predict these anomalies, we expect that both magnitudes are exactly equal to zero, and the red cross shows that our data support this hypothesis. On the other hand, we are able to exclude with high confidence all the magnitudes for these anomalies that are not included within the red ellipse. If these new effects actually exist, their signal in the CMS experiment cannot be larger because otherwise, we would have detected them.
Using advanced machine-learning techniques allowed us to achieve significantly higher precision than if we had used simpler algorithms. This result is part of a large CMS effort that paves the way towards the widespread use of artificial intelligence to help physicists make the most out of the very rich and intricate data produced by the LHC accelerator.
Read more about these results:
-
Do you like these briefings and want to get an email notification when there is a new one? Subscribe here